Tianyang Hu
Incoming Assisstant Professor, School of Data Science, CUHK-Shenzhen.
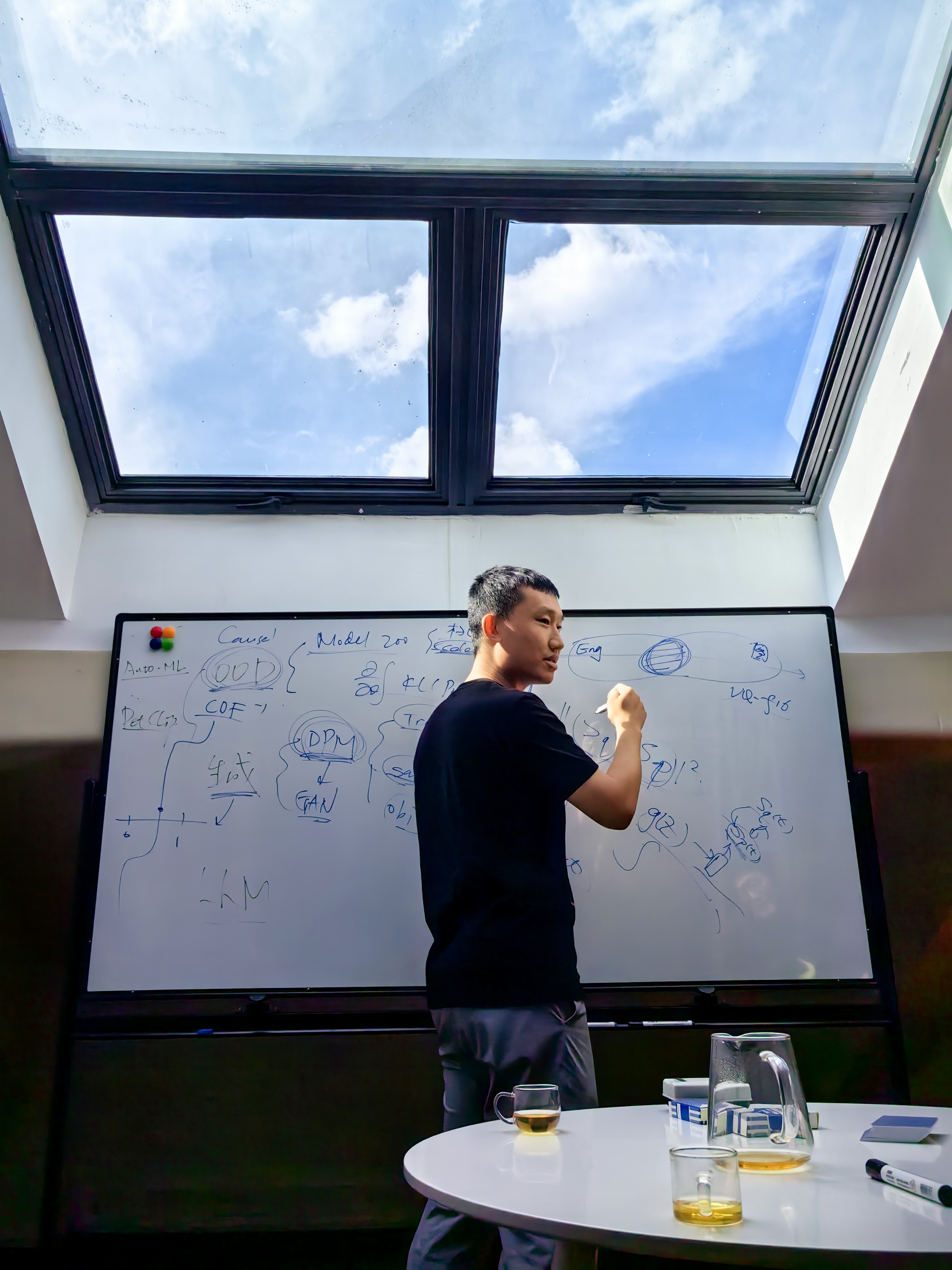
Photo taken in 2023 at SUFE, thanks to Yixuan and Taiyun :)
I am currently a Postdoc at the National University of Singapore, working with Prof. Kenji Kawaguchi. In Fall 2025, I will join the Chinese University of Hong Kong, Shenzhen as an Assistant Professor.
Previously, I was a research scientist at the AI Theory Group of Huawei Noah’s Ark Lab. I earned my Ph.D. in Statistics from Purdue University, under the supervision of Prof. Guang Cheng. Prior to that, I completed my M.S. in Statistics from the University of Chicago and B.S. in Math from Tsinghua University.
My research interests lie in the intersection of Statistics and AI, with the aim of advancing the theoretical understanding of AI and developing theory-inspired new algorithms. I am particularly interested in:
- Statistical Machine Learning
- Representation Learning
- Deep Generative Modeling
I am actively seeking PhD/MPhil students and research assistants with a strong theoretical foundation and hands-on experience in AI to join my group. If you are interested, please send me an email with your CV.
news
Nov 11, 2024 | I started a postdoctoral position at the National University of Singapore, working with Prof. Kenji Kawaguchi. |
---|---|
May 06, 2024 | 4 recent papers related to diffusion models are accepted, 2 on efficient sampling and 2 on training-free conditional guidance; I am now shifting towards understanding LLMs and my first work on the duality between prompting and finetuning is accepted by ICML 24! |
Apr 10, 2024 | Invited talk on the Duality Between Prompting and Weight Update in Transformers @ 2nd Workshop on ML Theory & Practice, Renmin University of China. |
Nov 25, 2023 | I will be hosting a session on AI4Math and Understanding LLMs in the 2023 X-AGI conference. |
Sep 22, 2023 | Two papers on generative modeling are accepted to NeurIPS 2023, including one Spotlight 🔦 |
selected publications
- ICMLExact Conversion of In-Context Learning to Model Weights in Linearized-Attention TransformersInternational Conference on Machine Learning, 2024
- ICMLReferee Can Play: An Alternative Approach to Conditional Generation via Model InversionInternational Conference on Machine Learning, 2024
- NeurIPS
Spotlight Complexity Matters: Rethinking the Latent Space for Generative ModelingAdvances in Neural Information Processing Systems, 2023 - NeurIPSDiff-Instruct: A Universal Approach for Transferring Knowledge From Pre-trained Diffusion ModelsAdvances in Neural Information Processing Systems, 2023
- JMLRRandom Smoothing Regularization in Kernel Gradient Descent LearningJournal of Machine Learning Research, 25(284), 2024
- ICLRYour Contrastive Learning Is Secretly Doing Stochastic Neighbor EmbeddingInternational Conference on Learning Representations, 2023
- NeurIPS
Spotlight Understanding Square Loss in Training Overparametrized Neural Network ClassifiersAdvances in Neural Information Processing Systems, 2022 - AISTATSRegularization Matters: A Nonparametric Perspective on Overparametrized Neural NetworkIn International Conference on Artificial Intelligence and Statistics, 2021
- JMLRMinimax Optimal Deep Neural Network Classifiers Under Smooth Decision BoundaryJournal of Machine Learning Research, Accept after minor revision, 2024